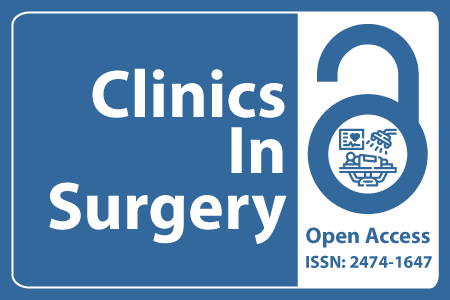
Journal Basic Info
- Impact Factor: 1.995**
- H-Index: 8
- ISSN: 2474-1647
- DOI: 10.25107/2474-1647
Major Scope
- Colon and Rectal Surgery
- Robotic Surgery
- Vascular Surgery
- Surgical Oncology
- Pediatric Surgery
- Transplant Surgery
- Oral and Maxillofacial Surgery
- Otolaryngology - Head and Neck Surgery
Abstract
Citation: Clin Surg. 2019;4(1):2344.Research Article | Open Access
Deep Survival Model Identifies Prognostic Subgroups of Triple-Negative Breast Cancer Patients
Isaac Kim, Sung Wook Seo, Jeong Eon Lee, Hee Jun Choi, Jai Min Ryu, Se Kyung Lee, Jong Han Yu, Seok Won Kim and Seok Jin Nam
Department of Surgery, Sungkyunkwan University School of Medicine, Republic of Korea
Department of Orthopedic Surgery, Sungkyunkwan University School of Medicine, Republic of Korea
*Correspondance to: Sung Wook Seo
PDF Full Text DOI: 10.25107/2474-1647.2344
Abstract
Objective: The aim of this study was to develop predict model for survival of BC patients using deep learning method and to apply our model for classifying the prognostic subgroups of TNBC patients.
Methods: We enrolled 7915 patients above pathologic stage 1 among 16254 patients who underwent surgery for breast cancer between May 1996 and January 2015. The enrolled patients were randomly sorted patients into a training group (80%, n=6288) and a test group (20%, n=1627). The Survival Recurrent Neural Network (SRN) model was constructed based on logistic regression function and long short-term memory recurrent neural network.
Results: The mean area under the receiver operating characteristics curve (AUC) of validation sets was 0.780 at 1st year, 0.840 at the 2nd year, 0.857 at 3rd year, 0.869 at 4th year, and 0.834 at 5th year. The mean AUC of test set was 0.945 at 1st year, 0.861 at 2nd year, 0.834 at 3rd year, 0.825 at 4th year, and 0.805 at 5th year. The c-index of the final model was 0.810 in the test group. In addition, the precision of SRN model was evaluated for classifying TNBC patients in test group. TNBC patients were classified into subgroups by SRN-suggested survival probability. Especially in pathologic stage 3 patients, SRN guided subgroups A, B and C were more precisely classified (P value <0.0001) than conventional stage IIIA, IIIB and IIIC (P value =0.292).
Conclusion: Our SRN model provides a useful tool for survival prediction of BC patients and also divides subgroup according to survival in TNBC patients.
Keywords
Breast Neoplasm; Machine Learning; Survival
Cite the article
Kim I, Wook Seo S, Eon Lee J, Jun Choi H, Min Ryu J, Kyung Lee S, et al. Deep Survival Model Identifies Prognostic Subgroups of Triple- Negative Breast Cancer Patients. Clin Surg. 2019; 4: 2344.